Supply Chains and Fuzzy Demand
Risk in supply chains can be included in several different ways into the decision making process.
No Risk
A statement in many supply chain models is that some/most/all parameters of the model are fixed (e.g. fixed demand, zero probability of a hurricane).
The result is, if the real value of this parameter diverges from the assumptions, the results of the model will be flawed to a certain degree (up to completely unusable).
Distributions
Another allegedly more complete way of including risks into modeling is to assign specific probabilities or even stochastic distributions the model’s parameters. In this way using a normal distribution, would represent the uncertainty about the future values of the parameter. Nonetheless, this approach has theoretical and practical flaws as well. From a practical standpoint, the time needed for solving the resulting model (especially with realistic model sizes) increases dramatically and may even be insolvable. And from a theoretical point of view, the assigned distributions may also prove to be wrong in reality.
Fuzzy Sets
Fuzzy logic is another way of introducing risk considerations into a model. This article is about the theoretical application of this logic to a supply chain problem.
Fuzzy sets are a construct which can be used to a limited amount of uncertainty into a system’s model.
A fuzzy demand variable, as an example, could have four possible manifestations of (14, 16, 18, 19). This can be interpreted as the demand can have one of those values without further specification of the individual probabilities.
Model and Results
Wen and Iwamura (2008) developed a facility location allocation model where the demand parameter has been defined as a fuzzy variable.
Since the demand in this case is not deterministic anymore, decisions have been based on an expected value.
The authors used the Hurwicz criterion, a decision function based on the weighted average of the worst and best outcome of a given decision, as the objective function to evaluate the “best” location-allocation.
They also suggest and test an solution heuristic. A possible solution of this location problem is shown in figure 1.
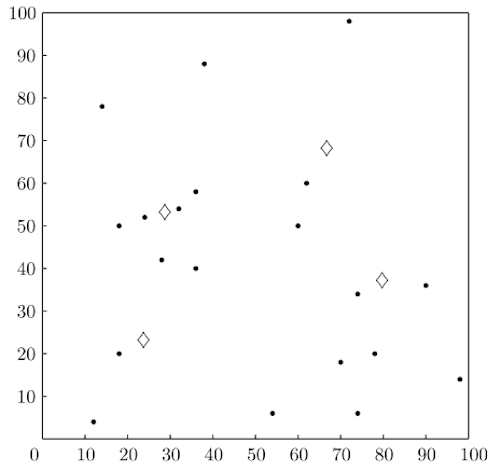
Conclusion
One advantage of the concept of fuzzy sets is that it may be easier for an analyst or expert to specify a limited number of possible demand values than fill the parameters an appropriate distribution function.
The disadvantages lie in the further assumptions of the fuzzy sets.
- The fuzzy variables are defined to be trapezoidal, ie. probabilities are assigned to the possible manifestations eg. (14, 16, 18, 19) in such way that the probability for the extreme values are lower than the center values. And this is basically the representation of a discrete distribution function.
- There is no evidence for fuzzy logic as better representing the uncertainty in a real supply chain system
- The data shows that the computational efficiency does not seem to be very good
Furthermore, fuzzy logic does not allow to represent extreme and unlikely events in a adequate manner. In the case of demand, there may be the possibility of extreme high or low demand which could lead to a change in the optimal locations.
Wen, M., & Iwamura, K. (2008). Fuzzy facility location-allocation problem under the Hurwicz criterion European Journal of Operational Research, 184 (2), 627-635 DOI: 10.1016/j.ejor.2006.11.029
Add new comment